Python Data Science Development
IPA-P102
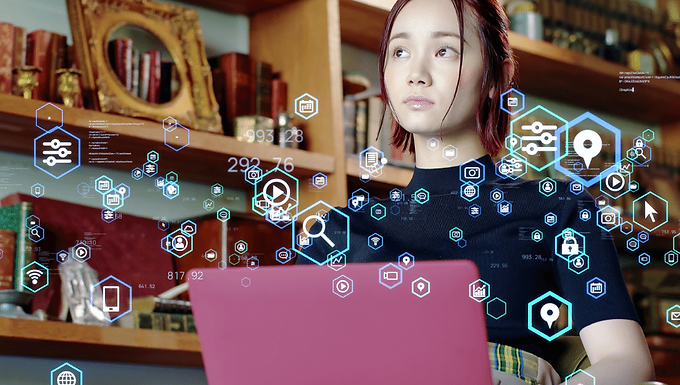
The IPA-P102: Python Data Science Development training program discuss the latest machine learning algorithms while also covering the common threads that can be used in the future for learning a wide range of algorithms. The course is a complete package that will help learners build their Python coding skillsets to meet the demand of the ML-AI industry which is growing by leaps and bounds in recent years.
Training Duration: 5 Days
OVERVIEW
Machine Learning, Artificial Intelligence and Deep Learning training program discusses the latest machine learning algorithms while also covering the common threads that can be used in the future for learning a wide range of algorithms. The course is a complete package that will help learners build their skillsets and meet the demand of the ML-AI industry which is growing by leaps and bounds in recent years.
This course on Machine Learning, Deep Learning and Artificial Intelligence goes beyond the theoretical concepts of the technology like regression, clustering, classification, etc. and discusses their applications as well.
OBJECTIVES
You will learn:
Introduction to Machine Learning, Artificial Intelligence, and Deep learning
Supervised and unsupervised learning concepts and modelling
Solving business problems using Artificial Intelligence and Machine Learning
Machine Learning algorithms
PREREQUISITES
Participants in this Machine Learning online course should have:
Familiarity with the fundamentals of Python programming
AUDIENCE
There is an increasing demand for skilled machine learning engineers across all industries, making this Machine Learning certification course well-suited for participants at the intermediate level of experience. We recommend this Machine Learning training course for the following professionals in particular:
Developers aspiring to be a data scientist or machine learning engineer
Analytics managers who are leading a team of analysts
Business analysts who want to understand data science techniques
Information architects who want to gain expertise in machine learning algorithms
Analytics professionals who want to work in machine learning or artificial intelligence
Graduates looking to build a career in data science and machine learning
Experienced professionals who would like to harness machine learning in their fields to get more insights
COURSE MODULES
Module 1: Introduction to Artificial Intelligence and Machine Learning
Artificial Intelligence
What is Machine Learning?
Machine Learning algorithms
Supervised Versus Unsupervised Learning
Machine Learning Algorithms
Regression
Classification
Clustering
Applications of Machine Learning
Machine learning examples
Setting up Anaconda & Python Notebooks.
Working on notebooks for Data Science
Module 2: Techniques of Machine Learning
Supervised learning
Unsupervised learning
Module 3: Mathematics & Statistics Refresher
Concepts of linear algebra
Euclidean and Non-Euclidean geometry
Introduction to Calculus
Probability, Conditional Probability, Bayes Theorem
Distributions, CDF, PDF
Mean, Median, Mode
Variance & Correlation,
Standard Deviation, quartiles, percentiles
Variable Relationships & Estimation
Hypothesis Testing
Module 4: Accessing/Importing and Exporting Data
Importing Data from various sources (Csv, txt, excel…etc)
Viewing Data objects
Exporting Data to various formats
Important python modules: numpy, pandas, scipy etc.
Module 5: Introduction to NumPy, Pandas
Create arrays using NumPy
Perform various operations on arrays and manipulate them
Indexing slicing and iterating
Read & write data from text/CSV files into arrays and vice-versa
Create Series and Data Frames in Pandas
Data structures & index operations in pandas
Importing and exporting data
Indexing and slicing of data structures in pandas
Reading and Writing data from Excel/CSV formats into Pandas
Module 6: Data Cleaning- Manipulation
Basic Functionalities of a data object
Merging of Data objects
Concatenation of data objects
Types of Joins on data objects
Exploring a Dataset
Analysing a dataset
Data Manipulation steps (sorting, filtering, duplicates, merging, appending, derived variables, sampling, Data type, conversions, renaming, formatting etc)
Data manipulation tools (Operators, Functions, Packages, control structures, Loops, arrays etc)
Python Built-in Functions (Text, numeric, date, utility functions)
Normalizing data
Formatting data
Module 7: Data Analysts-Visualization
Introduction exploratory data analysis
Descriptive statistics, Frequency Tables and summarization
Univariate Analysis (Distribution of data & Graphical Analysis)
Bivariate Analysis (Distributions & Relationships, Graphical Analysis}
Creating Graphs-
Bar plot
Pie plot
Count plot
Line chart
Histogram
Boxplot
Scatter
Density
Violine Plot
Swarm plot
Distplot
Pair plot
Heatmap
Important Packages for Data Visualisations
Matplotllb
Seaborn
Plotly
Cufflinks
SUPERVISED LEARNING:
Module 8: Linear Regression
Introduction- Applications
Assumptions of Linear Regression
Building Linear Regression Model
Understanding standard metrics
(Variable significance, R-square/Adjusted R-square, Global hypothesis ,etc)
Assess the overall effectiveness of the model
Validation of Models
Interpretation of Results- Business Validation
Implementation on new data
Module 9: Logistic Regression
Introduction- Applications
Linear Regression Vs. Logistic Regression
Building Logistic Regression Model
Understanding standard model metrics
Confusion matrix, accuracy score
Standard Business Outputs
Interpretation of Results- Business Validation
Implementation on new data
Module 10: Time Series Forecasting
Introduction -Applications
Time Series Components (Trend, Seasonality, Cyclicity and Level) and Decomposition
Classification of Techniques
Basic Techniques- Averages, Smoothening, etc
Advanced Techniques- AR Models, ARIMA, etc
Module 11: Decision Trees
Decision Trees - Introduction-Applications
Construction of Decision Trees through Simplified Examples
Generalizing Decision Trees; Information Content and Gain Ratio; Dealing with Numerical variables; other
Measures of Randomness
Pruning a Decision Tree; Cost as a consideration
Decision Trees – Validation
Over fitting- Best Practices to avoid
Module 12: Ensemble Learning
Concept of Ensembling
Manual Ensembling Vs. Automated Ensembling
Methods of Ensembling (Stacking, Mixture of Experts)
Bagging (Logic, Practical Applications)
Random forest (Logic, Practical Applications)
Boosting (Logic, Practical Applications)
Ada Boost
Gradient Boosting Machines (GBM)
XGBoost
Module 13: Naive Bayes
Concept of Conditional Probability
Bayes Theorem and Its Applications
Naive Bayes for classification
Applications of Naive Bayes in Classifications
Module 14: Model Evaluation, Improvements & Performance Metrics
Data Split Practices
Cross Validation
K-Fold Validation
Confusion Matrix
ROC Curves
Mean Absolute/Square Errors & R-Square
Ensemble Learning & Model Stacking
Module 15: Kernel Learning
Support Vector Machines
Principal Component Analysis
Ridge Regression
Spectral Clustering
Module 16: Support Vector Machines
Motivation for Support Vector Machine &Applications
Support Vector Regression
Support vector classifier (Linear &Non-Linear)
Interpretation of Outputs and Fine tune the models with hyper parameters
Validating SVM models
Module 17: Unsupervised Learning: Segmentation
What is segmentation & Role of ML in Segmentation?
Clustering algorithms
Concept of Distance and related math background
K-Means Clustering, Elbow method
Hierarchical Clustering
Module 18: Natural Language Processing
What is NLP & How to solve NLP problems
NLP Feature Engineering & Modelling
How to process any raw data file
Tokenizing, remove stopwords, speech tagging
Stemming, Lemmatizing, CountVectorizer, Wordcloud
Build models for solving practical read world problems.
Module 19: Deep Learning - Artificial Neural Networks (ANN)
Motivation for Neural Networks and Its Applications
Perceptron and Single Layer Neural Network, and Hand Calculations
Learning In a Multi Layered Neural Net: Back-Propagation and Conjugant Gradient Techniques
Introducing & Using Tensorflow
Neural Networks for Regression
Neural Networks for Classification
Interpretation of Outputs and Fine tune the models with hyper parameters
Validating ANN models
Module 20: End to End ML Implementation and Use Case specific discussions