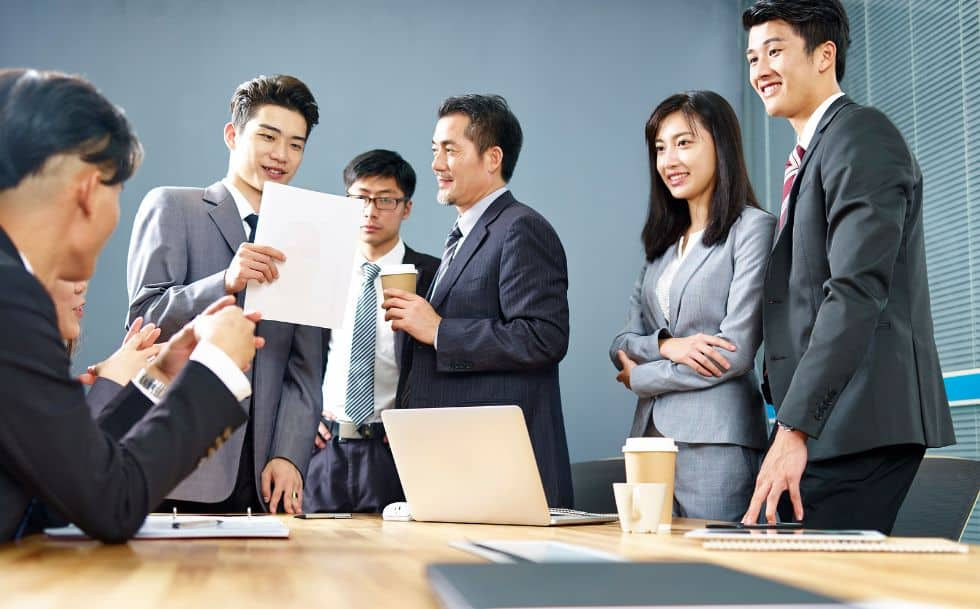
Join our training program to acquire the knowledge and skills needed to design and conduct data studies for informed organizational decisions and insights. Learn about business benefits, data evaluation methods, data pattern interpretation, project prioritization, complex data mining, statistical analysis, and more. Enhance your ability to measure team capabilities, extract valuable insights, manage projects, and communicate results. Gain expertise in analytics, data modeling, and the application of relevant tools. Unlock the potential of data science for your organization's growth and success.
Training Duration: 3 Days
- Certificate Of Completion Available
- Group Private Class
- VILT Class Available
- SBL-Khas Claimable
This programme is created specifically for learners who wish to design and conduct data studies to drive organisational decisions and insights.
- Organisational benefits of business insights
- Methods to evaluate data science solutions in contributing to efficiency, growth and return on investment
- Methods to identify and interpret the implications of data patterns
- Methods to prioritise proposed data science projects
- Methods to approach a business problem and come up with a solution that leverages the available data
- Methods to run complex data mining models
- Methods to visually and analytically explore a data set
- Methods to manage the capacity to perform data science projects
- Application of statistics, data mining and data modelling and the application of relevant tools and techniques
- Methods to measure the capability of the data science team
- Interpret implications of data patterns on business problem scenarios
- Exploit business data to extract insights
- Manage data science projects
- Configure and customise data models to investigate organisational business hypotheses
- Manage organisational capacity for performing data science projects
- Run complex data mining models to provide business insights in line with organisational procedures
- Communicate the results of data science projects
- Make recommendations to guide organisational decision making
MODULE 1: ADVANCING DATA ANALYTICS IN THE ORGANIZATION
Topics:- Introduction to Data Analytics for Business Intelligence professionals
- The big picture – big data and data analytics
- Overview of machine learning, data science, artificial intelligence and deep learning
- Discussion of analytics use cases
- Introduction to Analytics Project Life Cycle (APLC)
Mapped to:
- K1 Organisational benefits of business insights
- K9 Application of statistics, data mining and data modelling and the application of relevant tools and techniques
- A3 Manage data science projects
Rationale for Sequencing of the Units
- The first learning unit gives the learner an overview of big data, data analytics, machine learning, artificial intelligence and deep learning. They are brought through the different levels of analytics – descriptive analytics, diagnostic analytics, predictive analytics and prescriptive analytics.
- Learners are taught how analytics combines three schools of thought: Statistics, Machine Learning and Operations Research and are guided through an exercise on the situation present when the combinations of the three schools of thought are present. The analytics techniques, tools and application teaching examples include: visualisation for exploration), dashboard, clustering, prediction, network analytics, image analytics, video analytics and, modelling and simulation.
- Learners are introduced to Analytics Project Life Cycle (APLC), the various stakeholders, and how it can be used to manage data science projects. Learners have a group discussion on problem framing and data strategy (data components identification).
MODULE 2: INTERPRETATION OF DATA PATTERN VISUALLY
Topics:- Data patterns and shapes
- Data shapes interpretation on business
Mapped to:
- K3 Methods to identify and interpret the implications of data
- K7 Methods to visually and analytically explore a data set
- A1 Interpret implications of data patterns on business problem scenarios
- A2 Exploit business data to extract insights
Rationale for Sequencing of the Units
Subsequently, the next learning unit, it teaches the learners charts for data exploration and interpretation such as:- Relationship (scatter chart, bubble chart, network diagram)
- Comparison (column chart, bar chart, circular area chart, tornado chart, Pareto chart, multiple boxplot)
- Composition (pie chart, tree map, heat map, sunburst chart)
- Distribution (scatter chart, histogram map, bell curve, boxplot)
- Process (funnel chart, Sankey chart)
- Temporal (line chart, run chart, stacked column chart, stacked area chart, waterfall chart)
Learners are taught how to interpret implications on data patterns on business problem scenarios and exploit business data to extract insights.
MODULE 3: DESIGNING AN AUTOMATED AND USABLE DATA ANALYTICS PROTOTYPE
Topics:- Data science project data requirements, process and output data
- Data science result communication requirements
- Data model blueprint development
Mapped to:
- K5 Methods to approach a business problem and come up with a solution that leverages the available data
- K6 Methods to run complex data mining model
- K7 Methods to visually and analytically explore a data set
- K9 Application of statistics, data mining and data modelling and the application of relevant tools and techniques
- A4. Configure and customise data models to investigate organisational business hypotheses
- A6 Run complex data mining models to provide business insights in line with organisational procedures
- A7 Communicate the results of data science projects
Rationale for Sequencing of the Units
- In the next learning unit, the learners move to the next step in the sequence whereby they are brought through the reliability of data science outcomes. Learners are guided through a data supplies and input quality checklist, modelling process compliance checklist, planning, model training and model testing, model validation.
- Learners are taught about prototype to production to maintenance of data analytics then learners are invited to discuss issues such as: what data would be involved at production stage? How should the data be hosted or staged for scalability and efficiency of analytics service delivery?
- The learning unit concludes with the three key components of data science operation and sustainable data science.
MODULE 4: DATA SCIENCE ROI
Topics:- Quantify data science impact to business
- Total cost of investment of data science project
Mapped to:
- K2 Methods to evaluate data science solutions in contributing to efficiency, growth and return on investment
- K4 Methods to prioritise proposed data science projects
- A8 Make recommendations to guide organisational decision making
Rationale for Sequencing of the Units
- In the next learning unit, the learners are taught the quantifiable returns on data science initiatives such as growth, efficiency, productivity, and risk.
- Learners are guided through discussion topics such as, when would the value of data science initiatives be realized. And, is it possible that some returns cannot be calculated?
- Learners are brought through the total cost of investment of data science projects and to make recommendations to guide organisational decision making.
MODULE 5: ANALYTICS AS A SERVICE
Topics:- Service menu of data analytics team
- Capability and capacity of data and data science team
Mapped to:
- K8 Methods to manage the capacity to perform data science projects
- K10 Methods to measure the capability of the data science team
- A5 Manage organisational capacity for performing data science projects
Rationale for Sequencing of the Units
- The last learning unit teaches learners on providing analytics as a service.
- An entertaining kitchen analogy is used to make this topic relatable to learners to manage organisational capacity for performing data science projects.